PepsiCo leverages AI to create an intelligent monitoring system that improves Cheetos consistency
PepsiCo leverages AI to create an intelligent monitoring system that improves Cheetos consistency
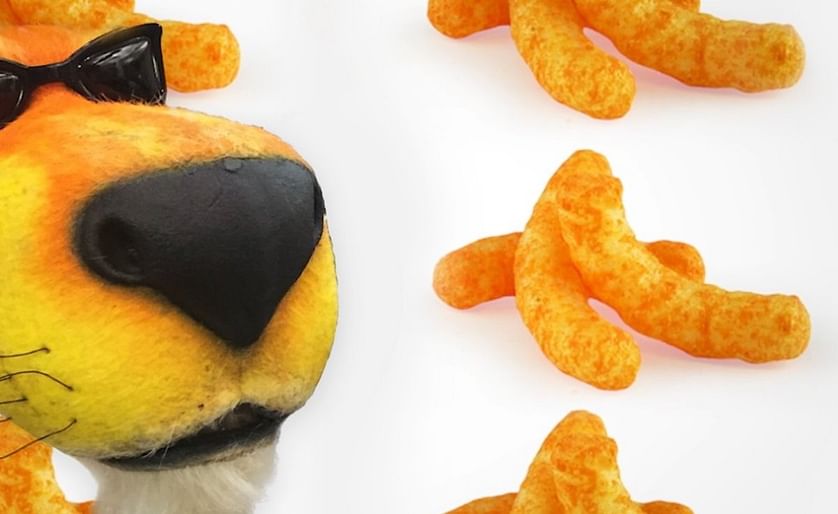
To the untrained eye (and mouth), a Cheetos Snack is a Cheetos Snack. But even die-hard snack lovers might not realize the lengths to which engineers go to ensure each bite has the optimal crunch, lightness, and shape.
PepsiCo, whose Frito-Lay division makes Cheetos and other beloved brands like Tostitos and Doritos, wanted a more efficient way to consistently manufacture Cheetos with the proper attributes while reducing waste.
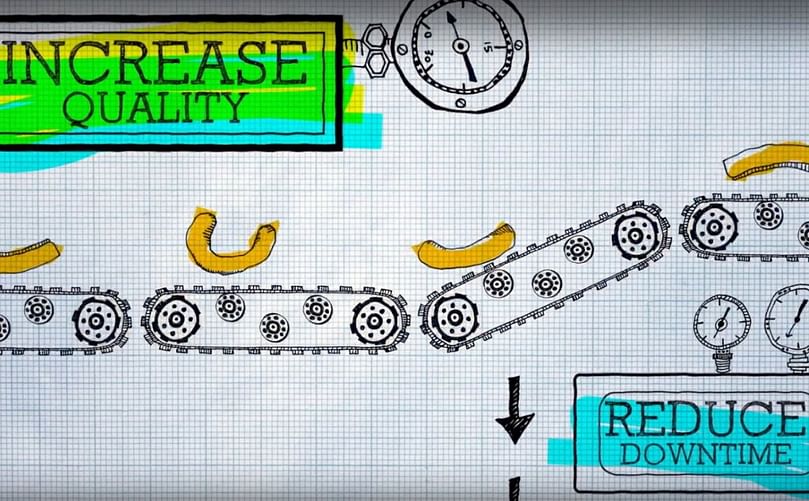
To meet this goal, PepsiCo developed an AI solution powered by Microsoft Project Bonsai that monitors and adjusts its extruders, the equipment that produces Cheetos.
The complex extruder was an ideal test case: Several inputs and specifications, including the ratio of cornmeal to water and the speed of the cutting tool, interact to create ideal Cheetos Snack characteristics.
The Project Bonsai solution has now proven itself at a pilot plant, where the technology does a good job of independently adjusting the extruder to maintain product quality and consistency.
Sean Eichenlaub, a senior principal engineer at PepsiCo:
“This is the future for process controls.”
“We're using AI-based automation to improve the consistency of our products.”
PepsiCo built a computer vision system that continually monitors Cheetos attributes.
Data about qualities such as density and length are fed to the Project Bonsai solution, which makes adjustments to bring the product within spec.
This approach reduces the time it takes to correct inconsistencies and allows operators to focus on parts of the line that require human expertise.
Kevin Lin, associate principal engineer at PepsiCo:
“Project Bonsai allows us to utilize technology for the things it does best and our employees for the things they do best.”
“The two complement each other and so far, we’ve seen great results.”
PepsiCo is preparing to use the solution in a production plant and exploring how to use the solution with other products, including the tortilla chip manufacturing process.
Denise Lefebvre, Senior Vice President of Global Foods R&D at PepsiCo:
“Innovation is a key ingredient in our success at PepsiCo and helps us deliver exciting new products, technology advancements and even new ways of working—whatever it takes to ensure we continue to bring smiles to our consumers every day.”
“Cheetos, one of our most beloved billion-dollar brands, are produced in 22 countries and come in more than 50 flavors.”
“The Project Bonsai technology helps us ensure each Cheetos Snack is perfect, and we’re excited about its potential. This is only the beginning.”
Innovation on the factory floor
An out-of-spec product can't be sold, which leads to wasted resources, time, and money. Greater consistency helps PepsiCo maintain high-quality products while maximizing throughput.
As part of the company's digital transformation efforts, PepsiCo began investigating how to apply the next generation of AI in its own operations a few years ago.
Project Bonsai empowered the company's experts to train the AI agent to operate successfully in a complex environment.
Dylan Dias, CEO of Neal Analytics, which partnered with PepsiCo and Microsoft on the project:
“Microsoft Project Bonsai offers a powerful approach to autonomous system design and implementation.”
“The project brought together a mix of technology, applied modeling skills, and subject matter expertise to create innovation on the factory floor.”
Training an algorithm live in the plant was simply unfeasible—PepsiCo couldn't risk an AI solution compromising safety or production schedule. That's one reason the Project Bonsai solution first learns in an environment carefully crafted to simulate the extruder line.
Developers worked with operators to re-create the conditions and reactions workers see in real life. The closer the simulator is to the actual line, the fewer adjustments the team will need to make before the solution can operate in the plant.
Jayson Stemmler, a technical project manager at Neal Analytics who worked on the PepsiCo pilot project:
“Subject matter experts give us an overview of how the process actually works in the plant.”
“They help us understand it every step of the way so we can build a simulator to replicate the extrusion line as closely as possible.”
Feedback reinforces learning
And instead of letting an AI solution learn what to do and not to do by trial and error alone, as conventional machine learning does, reinforcement learning builds positive and negative feedback. The difference between the two approaches can be compared to learning to ride a bike.
If you're left to your own devices, you'll probably figure out how to balance, pedal, and steer. But if someone is there to cheer on what you're doing right and point out your mistakes, you'll learn faster—and with fewer skinned knees.
Jayson Stemmler:
“When the platform reaches the Cheetos qualities we're aiming for, it gets the optimal reward.”
“In this way, the reinforcement learning algorithm learns what to do and what not to do while it's still in a simulation.”
Reinforcement learning means the solution can ultimately make recommendations even in conditions it didn't encounter in a simulator.
Jayson Stemmler:
“This solution comes to understand that “making an adjustment to one thing, and interactions between multiple adjustments, may have downstream effects.”Another benefit of starting with a simulation is that the solution can simulate a day's run in 30 seconds.
“These complex relationships are more easily captured by deep reinforcement learning, which is why this solution is more robust and able to learn better than a traditional model.”
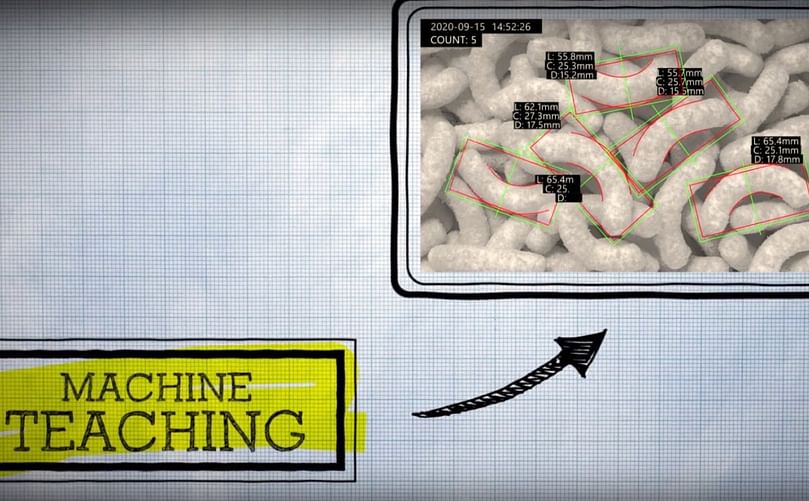
The algorithm learns the different combinations of adjustments that lead to the defined quality parameters in a fraction of the time.
Based on guidance from experts, developers also programmed rules necessary for safety. For example, the solution incrementally adjusts controls such as the rate a screw pushes cornmeal through the die, as changing the rate too abruptly could damage the machine.
The team also wrote code that prevents the solution from acting on a suggestion if the combination of adjustments could jam the machine.
These safety controls are one more reason operators are critical to designing such a solution.
Once the autonomous system solution had absorbed plenty of study material and was performing well within the simulation, it was time to see how it did in a test plant.
AI in action
To make an ideal Cheetos Snack, the solution needed examples of what wasn’t ideal – and needed to know what to do in those cases.
The extruder line is self-contained and well-suited for developing and testing an autonomous system solution. Operators had been running it manually, which gave developers the opportunity to build the solution from scratch, instead of on top of other software.
The AI solution has a recommendation mode and a closed-loop control mode. In both modes, a computer vision system continuously measures the quality of the Cheetos.
In recommendation mode, the AI will alert an operator if the product drifts out of spec, displaying on an instrument panel the attributes that are not ideal as well as a recommendation to correct it. The operator can push a button to make any or all recommended adjustments.
In the control mode, the only difference is that the AI solution skips the recommendation step and adjusts the extruder line specifications independently.
The company expects that running this intelligent control system will return the product to acceptable attributes faster.
In the current extruder line, operators measure product attributes manually at defined intervals. If the Cheetos are out of spec, the operator makes adjustments based on guidelines or experience to return the product to acceptable quality.
The problem: Infrequent sampling meant that the line could be producing out-of-spec Cheetos for a longer period of time without anyone realizing it.
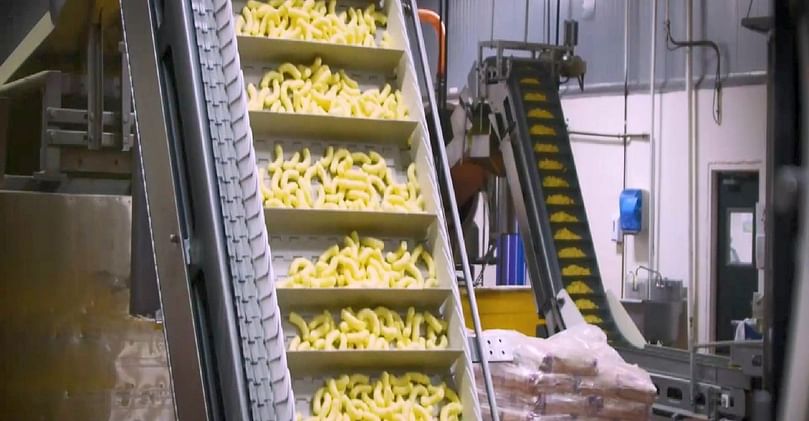
The Project Bonsai solution will monitor the product almost continuously, using sensors to oversee characteristics such as length and bulk density.
Also, the Project Bonsai solution learned from the company's most skilled operators, who worked as subject matter experts during the solution's training.
Jayson Stemmler:
“In this way, the brain could encapsulate the knowledge and skill of the best operators, then apply that through other facilities.”Working toward an independent AI solution
With the Project Bonsai platform, PepsiCo is translating its expertise in food manufacturing into an AI solution that adapts to changing environments.
Ultimately, PepsiCo aims to let this AI solution run autonomously. By empowering it to monitor the product and adjust the extruder line continuously and independently, the company expects to consistently maintain Cheetos quality and produce more throughput.
Dylan Dias:
“We are excited about our partnership with Microsoft and PepsiCo as we move forward to refine the intelligent agent and eventually deploy the solution, at scale, in factories and other industries around the world.”PepsiCo will continue to fine-tune the AI solution while preparing to extend it beyond the test plant and exploring other use cases.
Sean Eichenlaub:
“This extrusion line was our proof of principle.”
“Now that we've seen what Project Bonsai can do with one product, we are eager to find ways to improve processes across the entire company and product line.”